Pedram Akbarian
Email | GitHub | LinkedIn | Google Scholar
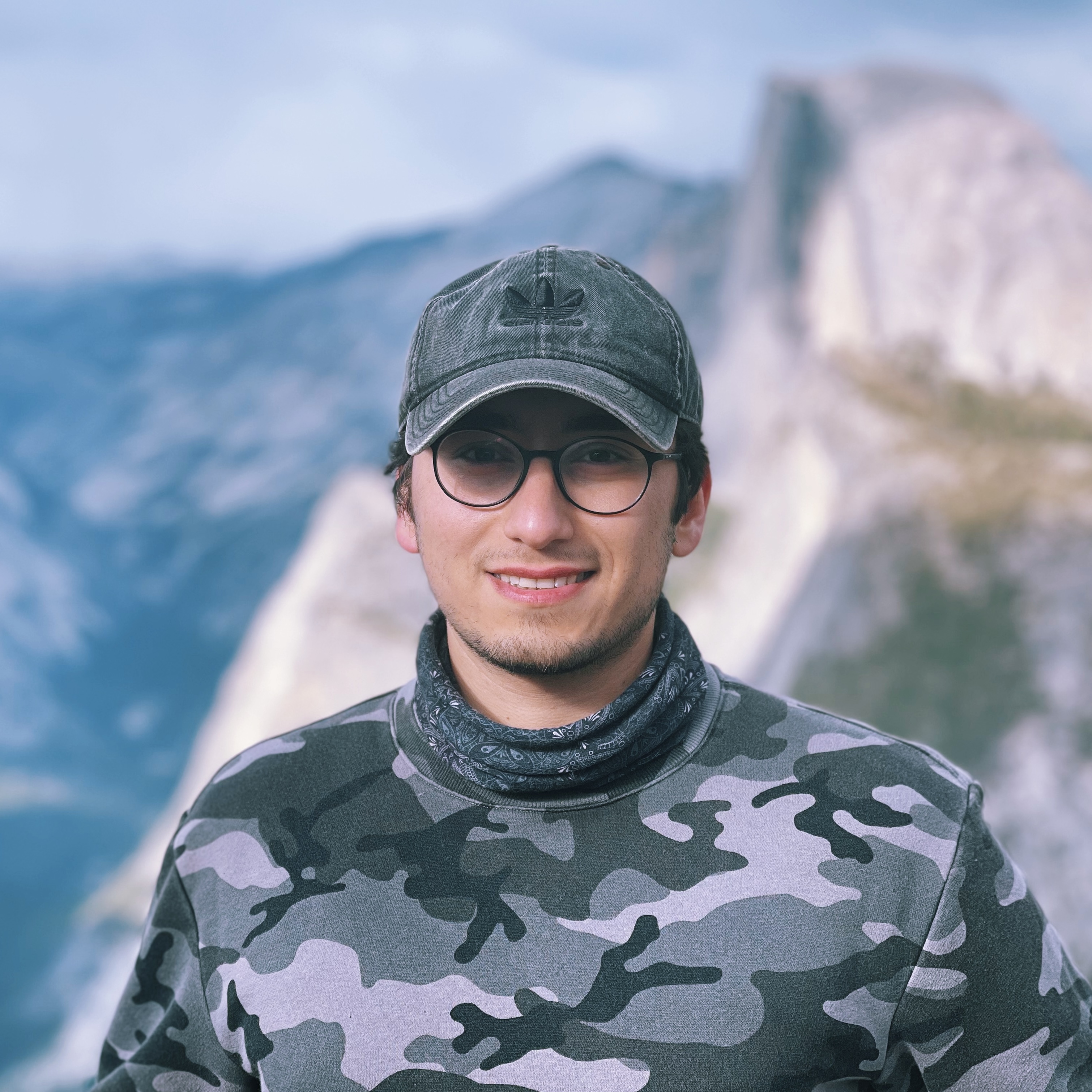
Email: akbarian@utexas.edu
I’m a PhD student in the Electrical and Computer Engineering Department at the University of Texas at Austin, advised by Prof. Nhat Ho and Prof. Atlas Wang. I’m broadly interested in theoretical and practical aspects of modern machine learning, with a focus on understanding the fundamental principles of designing and training scalable and efficient foundation models.
Currently, I am working on two primary research directions:
-
Efficient Training and Inference for Foundation Models: Focusing on statistical efficiency and training dynamics of Mixture of Experts (MoE) architectures to enhance scalability and performance in large foundation models.
-
Time Series Foundation Models: Exploring the fundamental limits and methodologies for developing scalable and generalizable models for time series analysis, with an emphasis on enhancing numerical reasoning capabilities.
Prior to joining UT Austin, I completed my Bachelor’s degree in Electrical Engineering with a minor in Computer Engineering at the University of Tehran, Iran. See my CV for more details.
selected publications
- PreprintSigmoid Self-Attention is Better than Softmax Self-Attention: A Mixture-of-Experts PerspectivearXiv:2502.00281, 2025Under review
- PreprintQuadratic Gating Functions in Mixture of Experts: A Statistical InsightarXiv:2410.11222, 2024Under review
- ICLRStatistical Advantages of Perturbing Cosine Router in Sparse Mixture of ExpertsIn International Conference on Learning Representations (ICLR), 2025
- ICMLImproving Computational Complexity in Statistical Models with Local Curvature InformationIn International Conference on Machine Learning (ICML), 2024
- ICMLIs Temperature Sample Efficient for Softmax Gaussian Mixture of Experts?In International Conference on Machine Learning (ICML), 2024
- ICMLA General Theory for Softmax Gating Multinomial Logistic Mixture of ExpertsIn International Conference on Machine Learning (ICML), 2024
- ICLRStatistical Perspective of Top-K Sparse Softmax Gating Mixture of ExpertsIn International Conference on Learning Representations (ICLR), 2024